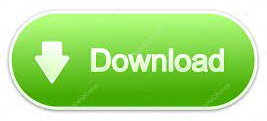
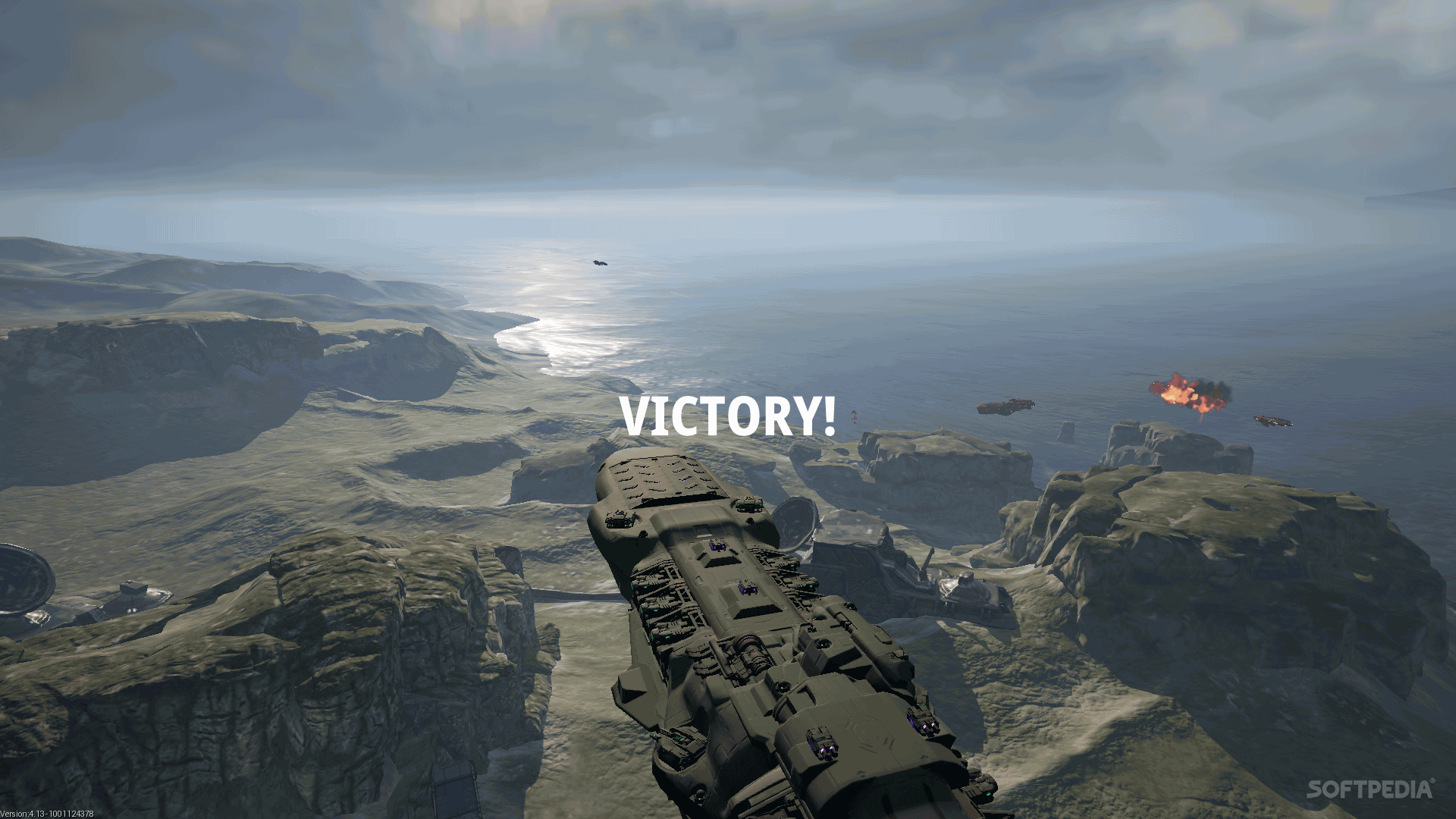

In contrast to the multifarious development of ironclads in preceding decades, the 1890s saw navies worldwide start to build battleships to a common design as dozens of ships essentially followed the design of the Royal Navy's Majestic class. The pre-dreadnought battleships were the pre-eminent warships of their time and replaced the ironclad battleships of the 1870s and 1880s.
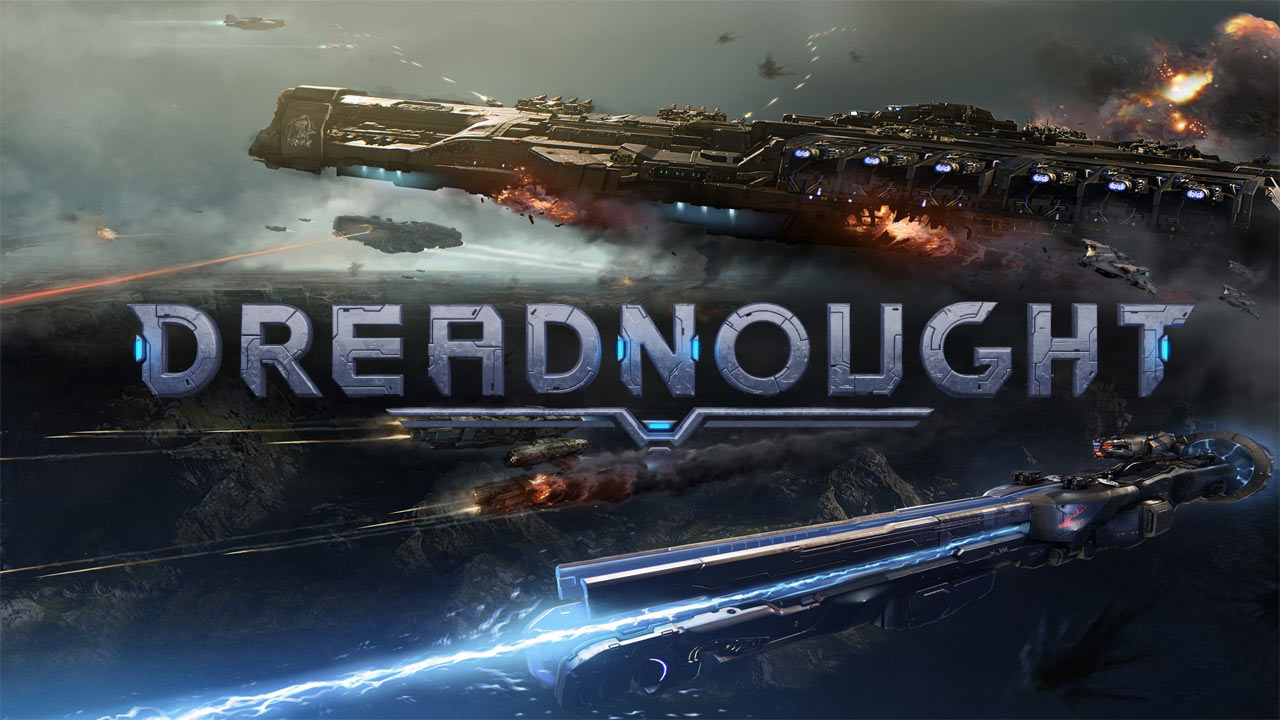
In their day, they were simply known as 'battleships' or else more rank-specific terms such as 'first-class battleship' and so forth. Their designs were conceived before the appearance of HMS Dreadnought in 1906 and their classification as 'pre-dreadnought' is retrospectively applied. Pre-dreadnought battleships were sea-going battleships built from the mid- to late- 1880s to the late 1900s. The improvement attained by prior works.HMS Royal Sovereign was the first pre-dreadnought battleship of the Royal Navy. That it is enough to apply image and text augmentations to make up for most of Training techniques that are popular in other subfields. Relative improvement on downstream zero-shot tasks, by using well-known That a simple CLIP baseline can also be improved substantially, up to a 25% Stronger training recipe is employed, the advantage disappears. That these baselines outperform a basic implementation of CLIP. In particular, we use the loss functions that were proven successfulįor visual self-supervised learning to align image and text modalities. Paper, we first propose, implement and evaluate several baselines obtained byĬombining contrastive learning with recent advances in self-supervised
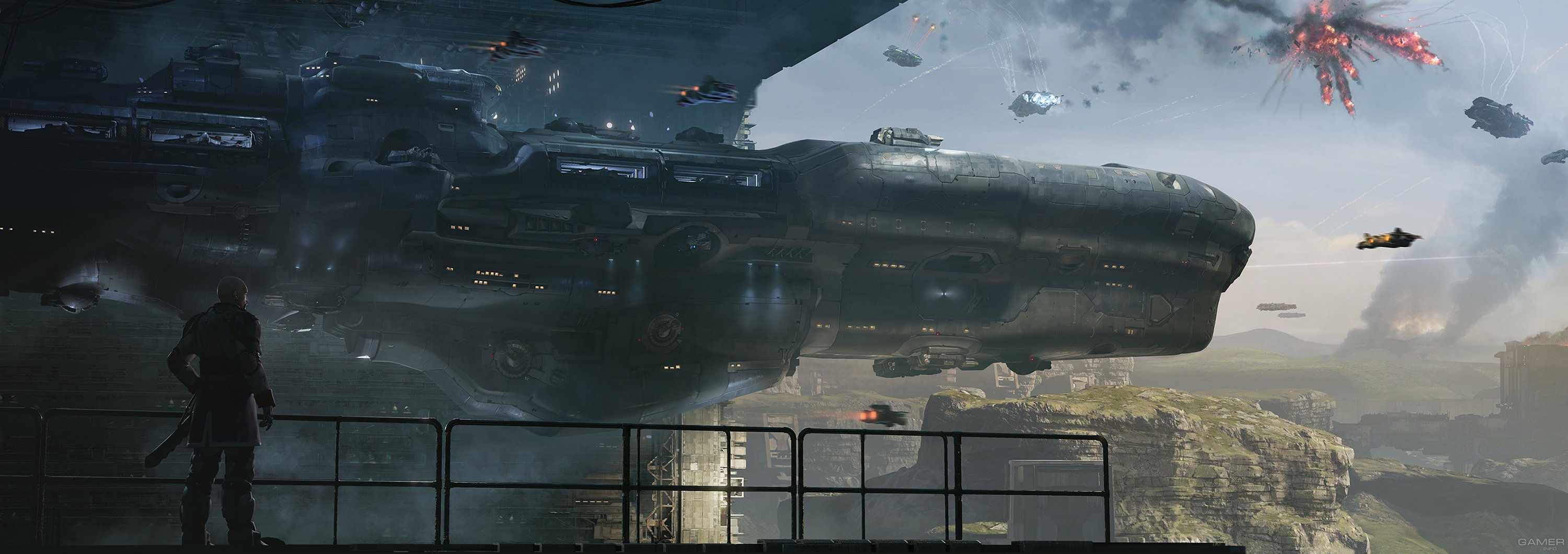
Other implementation details, e.g., data augmentation or regularization Sometimes hard to disentangle the contribution of these additional losses from Non-contrastive losses inspired from self-supervised learning. Recent work claims improvements over CLIP using additional Impressive results by training on paired image-text data using the contrastive CLIP, a seminal work in this area, achieved Download a PDF of the paper titled Improved baselines for vision-language pre-training, by Enrico Fini and Pietro Astolfi and Adriana Romero-Soriano and Jakob Verbeek and Michal Drozdzal Download PDF Abstract: Contrastive learning has emerged as an efficient framework to learn
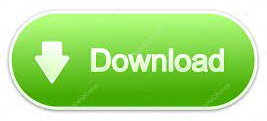